Machine Learning and Statistical Process Control (SPC) in Manufacturing
In the realm of artificial intelligence (AI), machine learning stands out as a dynamic subset that empowers computers to process intricate data, make informed decisions, and adapt autonomously to new information. Unlike conventional AI, machine learning doesn't just rely on preset rules; it learns from new data without manual intervention. This remarkable capability holds significant promise in managing large volumes of complex data generated by modern manufacturing processes.
A pivotal distinction between machine learning and statistical process control (SPC) lies in their data analysis capabilities. Machine learning excels at swiftly identifying anomalies and deriving insights from data, tasks that often take human analysts hours or days using SPC methodologies.
It's important to highlight that machine learning and SPC are not competing strategies but rather complementary tools that can coexist to optimize manufacturing processes. While SPC charts are effective at showcasing statistical control and in-process data, they can't replace the need for end-of-line (EOL) tests. EOL testing remains essential to ensure the quality of individual components. This underscores that SPC alone is insufficient in minimizing production waste and rework.
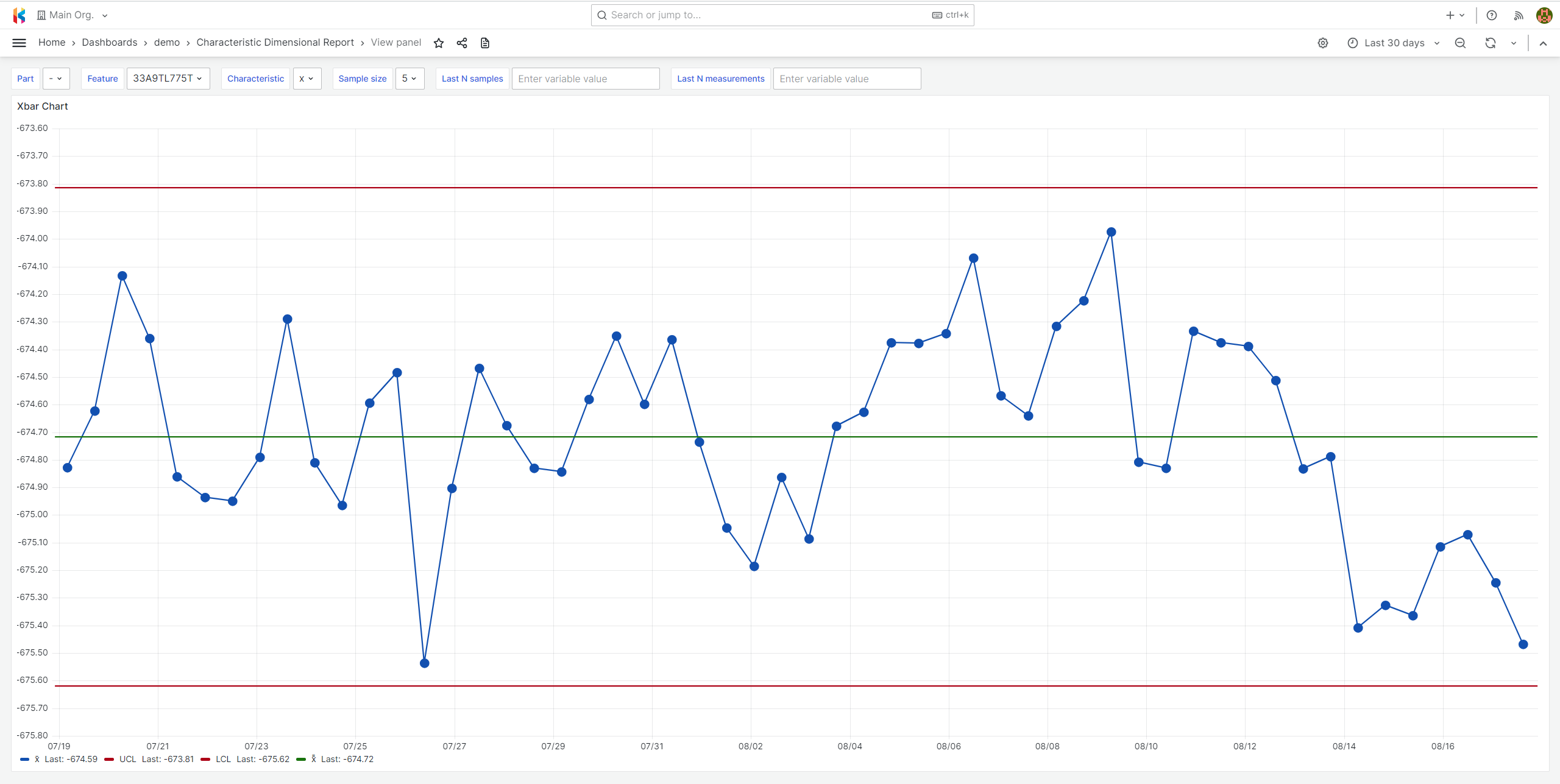
However, machine learning doesn't aim to supplant SPC; rather, it brings a fresh perspective to addressing manufacturing challenges. For instance, it can help answer confusing questions like, "Why do components fail EOL tests despite falling within specified limits?" While SPC involves statistical analysis of data trends, machine learning, delves into the intricate relationships between signals. This distinction allows machine learning to identify common abnormalities through a more holistic lens.
Consider an EOL test generating a staggering 1 000 000 time series data points. Here, machine learning truly shines by uncovering relationships within this vast dataset and comparing them across various transmissions to detect anomalies comprehensively. This level of complexity far surpasses manual analysis and even the capabilities of traditional SPC techniques.
Furthermore, machine learning's strength lies in its ability to collect actionable insights from interconnected signal relationships. This sets it apart from SPC, which predominantly focuses on the direction of single signal trends over time, lacking the capability to suggest solutions. Machine learning's ability in providing actionable insights transforms it into a valuable tool for effective problem-solving.
In the context of the automotive industry, SPC finds its niche in specific scenarios, such as monitoring features that intermittently impact downstream assembly. In such cases, SPC, combined with a well-defined sampling frequency, ensures the feature remains within statistical control. Conversely, machine learning offers a more comprehensive view of the entire manufacturing process, aiding in root cause analysis and providing holistic protection for the product's quality.
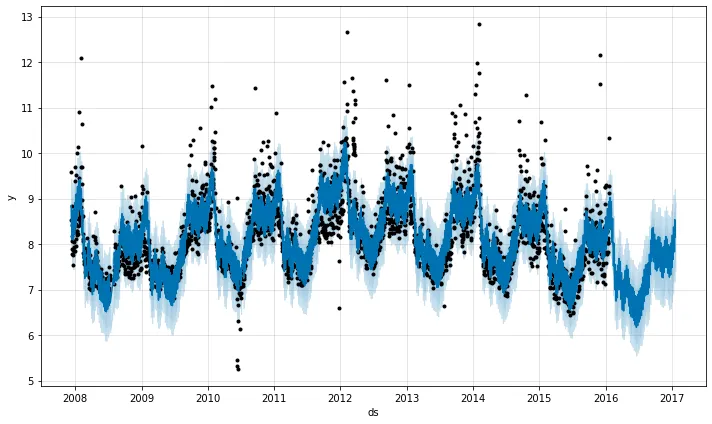
Machine learning steps into the manufacturing arena as a response to the surging volumes of data that modern plants generate. Just as the internet expanded the capabilities of computers, machine learning brings exponential possibilities to decipher patterns, connect seemingly disparate data, and present a comprehensive overview of production dynamics. Innovative solutions like KensoBI integrate SPC data with machine learning, heralding a new era of manufacturing analysis and quality management.
When conventional SPC proves inadequate in explaining downstream assembly issues despite favorable process checks, machine learning emerges as a tough ally. While SPC suffices for univariate data following a normal distribution, machine learning becomes indispensable when dealing with non-normally distributed multivariate data.
Machine learning's effectiveness hinges on the availability of extensive datasets, a common occurrence in modern manufacturing. Attempting to apply traditional statistical methods in such contexts overlooks inherent data imbalances. Moreover, the granularity of big data might amplify minor differences, making machine learning a more adept choice.
Beyond theoretical considerations, practical execution of SPC introduces variability due to human involvement. Operator-driven SPC, reliant on manual calculations and observations, can yield inconsistent results. In contrast, machine learning thrives on processed and visualized production line data, minimizing human errors.
In conclusion, the synergetic relationship between machine learning and SPC holds immense potential for enriching manufacturing insights. Machine learning's ability in unraveling complex relationships and patterns, coupled with its ability to provide actionable insights, fills the gaps left by SPC's limitations. As manufacturing experiences, a paradigm shift toward data-driven decision-making, integrating machine learning alongside SPC emerges as a pivotal strategy for optimizing processes, minimizing waste, and upholding product quality. Are you ready for the next generation of manufacturing analysis?